We've made huge leaps in tracking customer behaviour through online ads, writes ClickThrough digital content executive Oliver Pyper, but we still haven't found a foolproof way to track customers once they step away from the screen and visit a branch in person. In this post, he explores four options that go some way towards bridging this data gap.
20 years ago, a pensioner in Gateshead, Tyne and Wear, planted the seeds of an Internet revolution that would snowball into a multi-billion pound industry.
Appropriately enough, the pensioner’s name was Mrs Jane Snowball. In May 1984, this grey-haired grandmother became the first ever customer on the first ever B2C Internet shopping network.
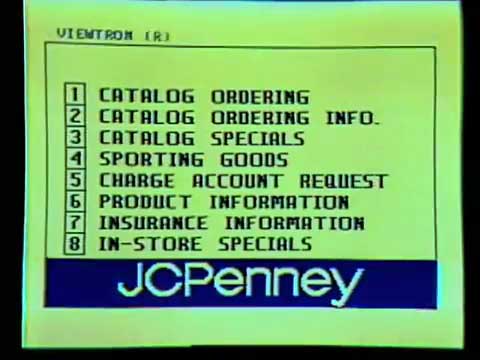
The world of e-commerce nowadays is a million miles away from the clunky ‘teleshopping’ experience that Mrs Snowball had to contend with. But for all the sophisticated analytics tools we use and our careful monitoring of ad spend, we still haven’t found a reliable way to track visitors who click on an ad, research online, then buy in-store. From our own online shopping experiences, I’m sure we can all vouch for the fact that this happens. A lot.
And with ever-surging rise of mobile and the need to track behaviour across multiple devices, the logistics of implementing such a system may seem more difficult than ever. However, mobile will almost certainly hold the key to unlocking this hitherto-undiscoverable data.
Before we dive in and find out why, let’s have a quick revision session.
Here’s where we are now:
- Phone call tracking is a reliable and effective way to track queries and purchases that originate online before switching to phone. However, this doesn’t help us track real-life, in-store footfall and purchases.
- Google AdWords lets you track conversions that originate online before moving in-store, but only in certain situations (see below).
- Google is trialling the use of anonymised purchase data from retail partners to test the effectiveness of online ads driving in-store sales. They haven’t mentioned exactly what form this technology might take.
The most important point is this: There is currently no fool-proof way to completely smooth over the cracks between online and offline. But there are
Approach One: Using Unique IDs and Collecting Customer Information
You can do this right now using Google AdWords.
Or rather, you can do this if your business collects enough customer data to make this approach viable.
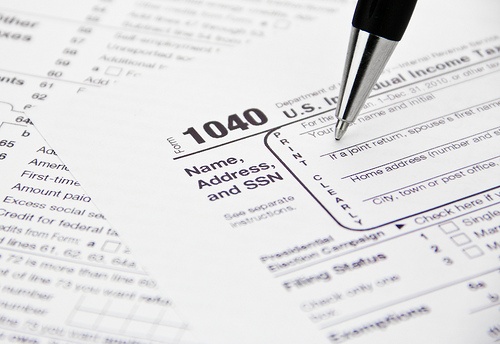
It works by assigning a unique ‘GCLID’ to each click you get from a paid search ad. This GCLID is then saved along with any customer data you can retrieve during their visit to your site.
When the customer completes a sale, or requests a quote, or otherwise meets conversion criteria, you upload this data from your CRM system to AdWords, where your data will be available for analytical purposes.
So it’s essentially an easy way of joining the dots between different sets of data, and letting you see this data within the AdWords interface.
It’s great if you’re the kind of business that relies on collecting lots of customer data throughout its sales process. But it’s not so useful at tracking conversions for retail businesses that rely on quick, -non-intrusive sales processes – like shoe stores, supermarkets, and so on.
These kinds of retailers demand a different approach.
Approach Two: Couponing
And here it is. In recent times, Google has been gently giving bricks and mortar businesses a bit of a break, implementing features in its ad offerings that focus on driving footfall in-store, rather than pushing online purchases exclusively.
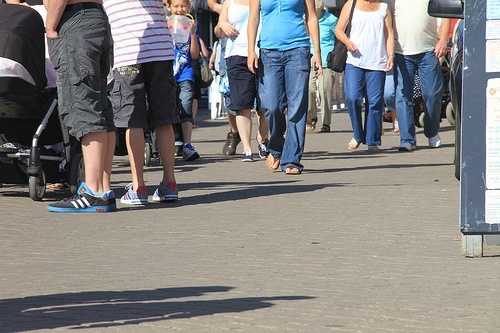
One example of this is Google Offers. These are special ads that only appear to customers that are searching within the vicinity of a retail location, and take the form of a coupon that can be saved to their smartphone and redeemed in store.
When the coupon is redeemed, the customer’s unique ID is recorded, so you can marry up their online activities with their ‘real-world’ visit.
This means those aforementioned retailers who rely on quick checkout purchases have a way of tracking customer behaviour from online to offline.
However, it goes without saying that this leaves huge gaps in your data. Chances are the vast majority of customers and their behaviours are still going unrecorded.
And you could take the data you collect through Google Offers and scale it to model the behaviour of your whole customer base. But the data you’ve collected will be naturally skewed towards the kind of customers who are likely to redeem coupons.
If we’re going to go down the route of modelling customer journeys from offline to online, we’re going to have to rely on a much more robust data set.
Approach Three: Testing the Impact of Marketing Actions on Footfall and Purchases
There are several ways to model in-store customer footfall and purchases based on online actions. We’ll look at two – A/B geo-testing and promoted categories.
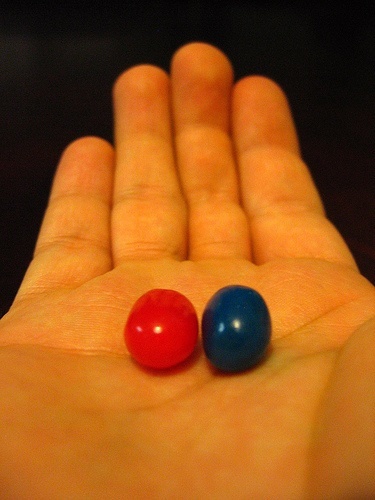
A/B geo-testing involves increasing mobile spend in certain parts of the country, and seeing if in-store visits and conversions increase in the areas with increased spend. Simple, but not very scalable.
Or, you can split things down by product category. Take two categories that are similarly affected by seasonal trends, and increase ad spend on one. If you see an uplift on in-store purchases for the promoted category, you can reasonably surmise that this was (at least partly) down to interest in your paid search ads.
These approaches can be extended beyond paid search. You can apply it to any online marketing action, whether a PR campaign or a content marketing push. However, the flexibility of AdWords analytics makes it particularly suited to PPC campaigns.
Depending on your business’s circumstances, the quality of the data you’ve got to work with, and whether fate decides to skew your results with some act of God or other, these modelling approaches can be a fairly reliable way to map the effect of online actions on offline conversions.
Approach Four: Wi-Fi Tracking
Before we examine this approach, let’s look at the things we’ve learned so far.
You can use Google AdWords IDs to track individual customer journeys, but for most businesses this leaves huge swathes of potential data untracked.
You can use modelling and A/B testing to test how online marketing actions affect offline conversions and visits, but this is all about the bigger picture – it doesn’t give you detailed insights into how each customer behaves.
The holy grail of online to offline conversion tracking would be an approach that provided in-depth insights on a wide scale.

The only way to do this is to track the digital and bricks-and-mortar behaviours of as many individuals as possible.
Short of passing a law that requires everyone to wear personal GPS devices, this might seem far-fetched. But thankfully, most of us carry something fairly similar around with us every day – smartphones.
By detecting the presence of Wi-Fi enabled smartphones, you can detect whether customers are in your store, and how they behave when they’re there. The technology exists to do this – it’s the basis of Walkbase’s business model for a start.
The data you can gather from technologies like Walkbase can be applied to the modelling methods we’ve already discussed to give you a much more useful data set. However, it doesn’t entirely solve our problem – it won’t give you online-to-offline behavioural data for each individual customer.
To do this, we’d need to ensure those unique AdWords IDs, or other useful pieces of personal information, were available to track in-store. To gather this data using the in-store tracking devices, the customer would have to be signed in on their mobile – on a retailer’s app, for example.
So, this is a hurdle in itself. But as the Internet of the Things continues its inexorable emergence into our everyday reality, we can assume things are going to get easier on this front.
But if you’re not ready to wait, you could speed the process along by actively rewarding the customer for being signed in when they’re in store. For example, by providing a really useful app that provides real-time information about products and offers in the store they’re in.